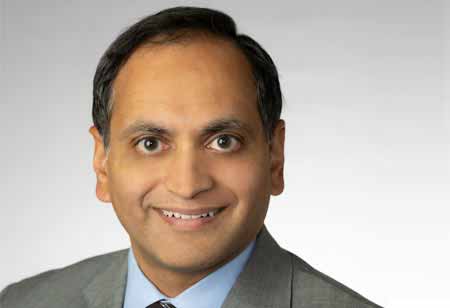
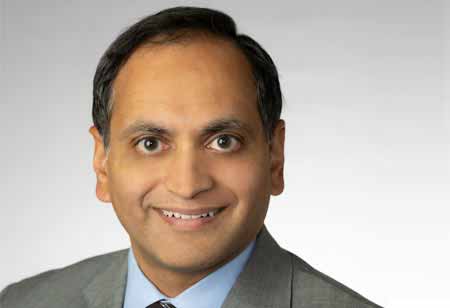
Thank you for Subscribing to Medical Care Review Weekly Brief
In oncology, what are the biggest challenges you face as new therapies and treatments come to market?
Rapid advancements in oncology require us to continuously adapt. As new therapies become available, we need to identify which patients are eligible for these new treatments. This increasingly depends on testing for the relevant biomarkers and supporting clinicians in staying up-to-date on the newest standards of care. As the co-chief medical officer for the medical oncology network at UPMC Hillman Cancer Center, in Pittsburgh, PA, we need to make sure eligible patients can access those drugs. What technological challenges do you see in addressing these challenges? Patient electronic medical record data contains unstructured documents and text, requiring human intervention to mine the laboratory and genomic data to determine whether that patient is eligible for the treatment. Ultimately, we need that data structured so that we can quickly utilize it in real time and analyze it continuously. We also need a trusted ecosystem where we can validate the output and measure the clinical performance against the standard of care. To that end, we work with precision medicine analytics company to organize and analyze the data. The analytics company provides the essential metrics that reflect the quality of care without the noise of more complex solutions and develops performance scorecards at the care site and provider level. How do you measure the adoption of new standards of care with your practice? We set up quality initiatives across various cancer types to stay on top of the newest standards of care. We set a baseline to evaluate how we are doing in the testing and treatment of those cancers. From there, we establish goals based on the results, determine what interventions we can make to address any gaps identified, and then review progress against those goals at our quarterly physician meetings. "In the future, I see a world where we can leverage AI and large language models to identify eligible patients for treatment ahead of time while using predictive analytics to identify those at risk for treatment failure or needing intervention." What will the next level of quality improvement code look like? Today, our quality initiatives are reactive such that we must look back later to see whether the patient received the test or treatment they should have. In the future, I see a world where we can leverage AI and large language models to identify eligible patients for treatment ahead of time while using predictive analytics to identify those at risk for treatment failure or needing intervention. The maturation of these AI and language tools would allow the clinician to be proactive, determining the best course of action even before the patient enters the office for care. How do you determine the effectiveness of these quality initiatives? We set a baseline based on past performance and monitor adherence to standard of care against that baseline over the next year. We also look at outcomes such as overall survival. We are interested in the standard of care and the impact we have on our patients. We expect to see that our post-baseline approach improve patient outcomes relative to the baseline period. In the future, we plan to integrate cost data to create a more comprehensive view to drive quality improvement and reduce the total cost of care.